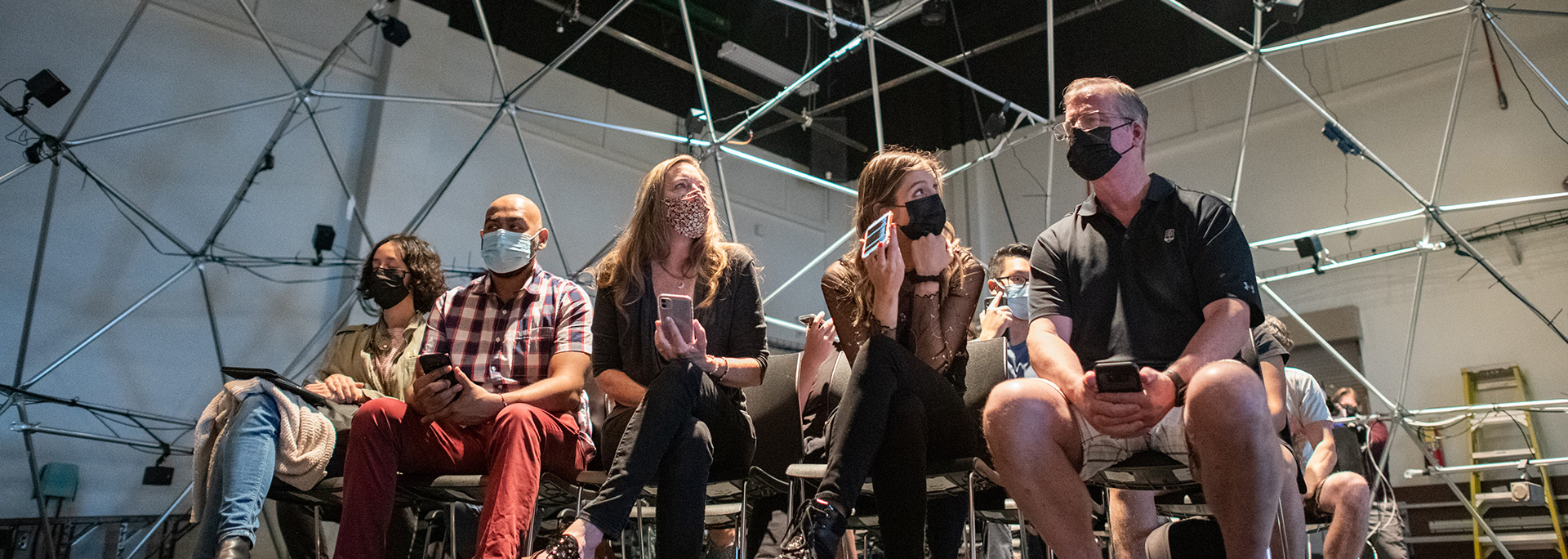
Events
The School of Arts, Media and Engineering hosts several events throughout the year.
Explore our events
From Fabrication Lab workshops to our Esports Lounge, we have something for everyone.
ASU Events
The School of Arts, Media and Engineering hosts several events throughout the year.
From Fabrication Lab workshops to our Esports Lounge, we have something for everyone.